Balancing innovation in the financial services sector with risks in data privacy, cybersecurity and regulatory compliance.
Banks are actively exploring use cases for generative AI. In fact, around 58% of banks report that they are currently evaluating or testing GenAI in some capacity, according to Celent’s ‘Harnessing the benefits of AI in payments’ report.
However, with great innovation comes great responsibility, particularly in highly regulated industries like FSI. It is essential to explore what financial service organizations can do to unlock the full potential of generative AI at scale while mitigating risks associated with data privacy and security breaches.
DigifinAsia sought out some insights from Stewart Garrett, Regional Vice President, ASEAN & Japan, MongoDB.
The financial services industry (FSI) has been using artificial intelligence (AI) for years. Why are companies doubling down on investments here in 2024?
Garrett: In Celent’s latest Technology Insight and Strategy Survey, 73% of corporate banks reported that they had delivered clear revenue opportunities from their investments in advanced data analytics, compared to 11% that reported the reverse. As a result, it is no surprise that projects supported by data analytics and artificial intelligence (AI) technologies are high on the agenda globally.
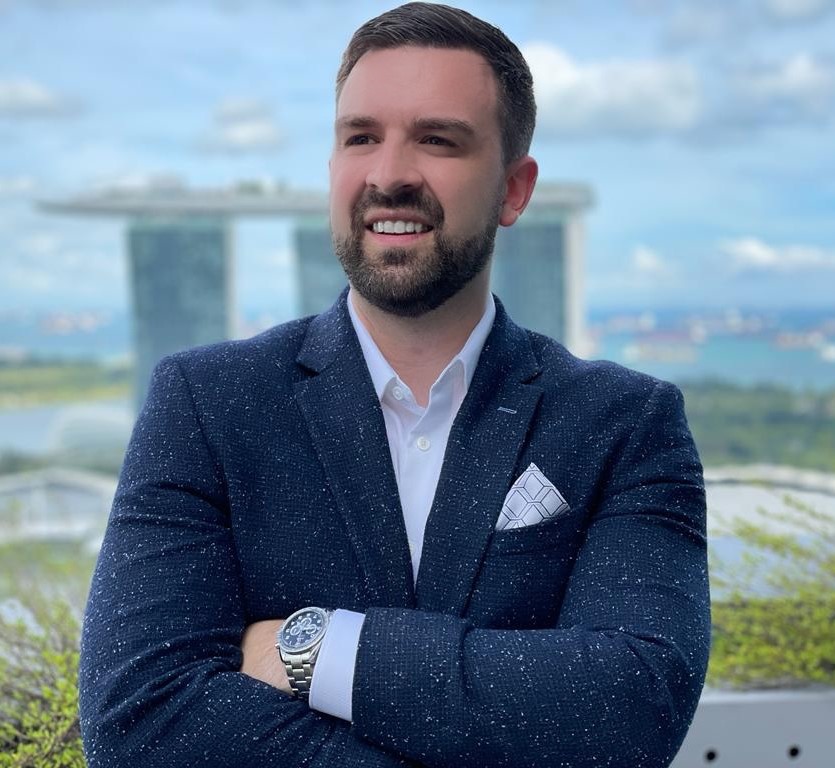
Advanced data analytics and AI initiatives present revenue opportunities across industries, including banking. Celent’s survey highlights this trend, with 30% of global banks increasing their spending on payment technology, ranking AI as a priority for the year ahead.
Rising consumer expectations over the speed and quality of digital services have led banks to double down on AI investments to enhance their operations and payment technology. For instance, Bendigo and Adelaide Bank, one of the most trusted brands in Australia, uses MongoDB Atlas and generative AI tooling to automate repetitive developer tasks. This enhances efficiency and the pace of innovation to improve end-user experiences. For example, it has reduced the time spent running application test cases from over 80 hours to just five minutes.
The conversation around generative AI became more nuanced through the latter part of 2023. This is understandable given the complexities of applying large language models (LLMs) to sensitive customer data, as well as broader regulatory concerns over the explainability of LLM outputs. That said, there are many areas in which generative AI is already being used to support advisors and relationship managers, with further innovation expected.
What are some of the emerging AI and generative AI use cases in payments?
Garrett: Many areas in the front office, middle and back-office functions can benefit from AI. Customer-facing teams can use AI to enhance or provide value-added services for customers, such as providing account insights to customers and supporting payment delivery, where customers can inquire about the status of their payments or bank accounts.
The use of AI and advanced analytics is already commonplace across large areas of the middle and back-office functions for payment processing, but AI can be used to further augment these areas. Machine learning can further automate the payment repair process, lowering the need for manual intervention. Doing so reduces costs for the bank while also improving the customer experience.
Another benefit of AI is risk and fraud detection. Natural language processing (NLP), computer vision, and machine learning can be used collectively to reduce the level of false positives, in turn freeing up costs and resources for manual review. Data can also be fed to AI-powered fraud detection models to identify potential risks and fraud attempts.
What capabilities do FSIs need to adopt these use cases at scale?
Garrett: Data-led services or other use cases for AI must not be treated as one-off initiatives or tactical product enhancements. Adopting AI at scale requires a long-term strategy and investments in the capabilities to support product enhancement and innovation.
This strategy can be broken down into three pillars.
- Modernizing payment infrastructure: While it may be possible to deliver service improvements on top of legacy technology, it will be easier, cheaper, and faster to unlock value when the basics in terms of process simplification and data structure are in place.
- Creating the right data architecture: Flexibility and performance will become increasingly important, and taking advantage of modern database technology, including harnessing the scalability of cloud services, should also be a priority for banks looking to future-proof their investments. Banks must ensure that their data architecture is flexible enough to allow the integration of multiple different data types, sources, and models.
- Skills, structure, and organizational culture: As organizations embark on incorporating data analytics and AI into their workstreams, employees across the organization must possess the right skill sets in areas such as machine learning, NLP, Gen AI, advanced analytics, and data science. A company’s culture has a strong impact on how quickly and successfully an organization can adopt AI, so banks must create a culture that highlights the importance of adopting new technologies and how they can drive innovation. Across all of this, creating a culture in which teams feel safe to experiment and potentially fail fast is also crucial.
How should FSIs balance AI innovation with privacy and security concerns?
Garrett: Organizations looking to strike the right balance between innovation, privacy, and security concerns must adopt a holistic approach consisting of competent data management platforms, as well as comprehensive data and AI governance frameworks.
It is essential to use a data management solution that is fast, flexible and offers robust security. A scalable and efficient platform like MongoDB drives innovation by allowing banks to enjoy deployment flexibility and scale according to demand. That is why Bendigo and Adelaide Bank chose MongoDB Atlas as the underlying platform for its tech stack because of its high levels of security and reliability and ability to manage the bank’s real-time operational data on a unified platform. MongoDB Relational Migrator – a tool that helps businesses eliminate barriers like time, cost, and risk frequently associated with cloud migrations – also enabled them to expedite codebase analysis, service decomposition, and application refactoring, ensuring a secure and efficient migration.
Having a comprehensive data governance framework in place also ensures that customers’ personal data is managed and protected in a responsible manner and that organizations are adhering to privacy regulations.
The next step would be to establish robust AI governance frameworks. This will comprise policies, processes, and controls for the ethical and secure deployment of AI across the organization. By monitoring and evaluating the algorithms, organizations can prevent the AI models from generating flawed decisions or results.
The following recommendations will help ensure financial services organizations can unlock the transformative potential of generative AI at scale while ensuring privacy and security concerns are adequately addressed:
- Train AI/ML models on the most accurate and up-to-date data, thereby addressing the critical need for adaptability and agility in the face of evolving technologies.
- Futureproof with a flexible data schema capable of accommodating any data structure, format, or source. This flexibility facilitates seamless integration with different AI/ML platforms, allowing financial institutions to adapt to changes in the AI landscape without extensive modifications to the infrastructure.
- Address security concerns with built-in security controls across all data. Whether managed in a customer environment or through a fully managed cloud service such as MongoDB Atlas, ensure robust security with features such as authentication (single sign-on and multi-factor authentication), role-based access controls, and comprehensive data encryption.
- Launch and scale always-on and secure applications by integrating third-party services with APIs. A flexible data model and the ability to handle various types of data, including structured and unstructured data, would be a great fit for orchestrating your open API ecosystem to make data flow between banks, third parties, and consumers possible.
What’s the first thing FSIs need to do to harness the benefits of AI?
Garrett: AI is still in a nascent stage across industries, including the financial services industry. However, some banks have begun advancing rather quickly. Identifying and working on AI-related areas of improvement and opportunities that are unique to each bank will be the difference between pulling ahead of the competition and falling behind.
There are many ways in which a bank can use AI technologies to enhance customer-facing services, or otherwise improve operational efficiency. Zooming into a particular area will allow banks to direct the right resources and allow for sufficient time for these improvements to take place. Some may focus on the immediate improvements that can be made to middle and back-office processes, while others will address more directly customer-facing areas.
Banks must recognize that there is no silver bullet that can help them leverage AI to significantly drive up revenue or profitability. However, they can position themselves for long-term growth by prioritizing their efforts and ensuring that they have the right foundation to support AI success.